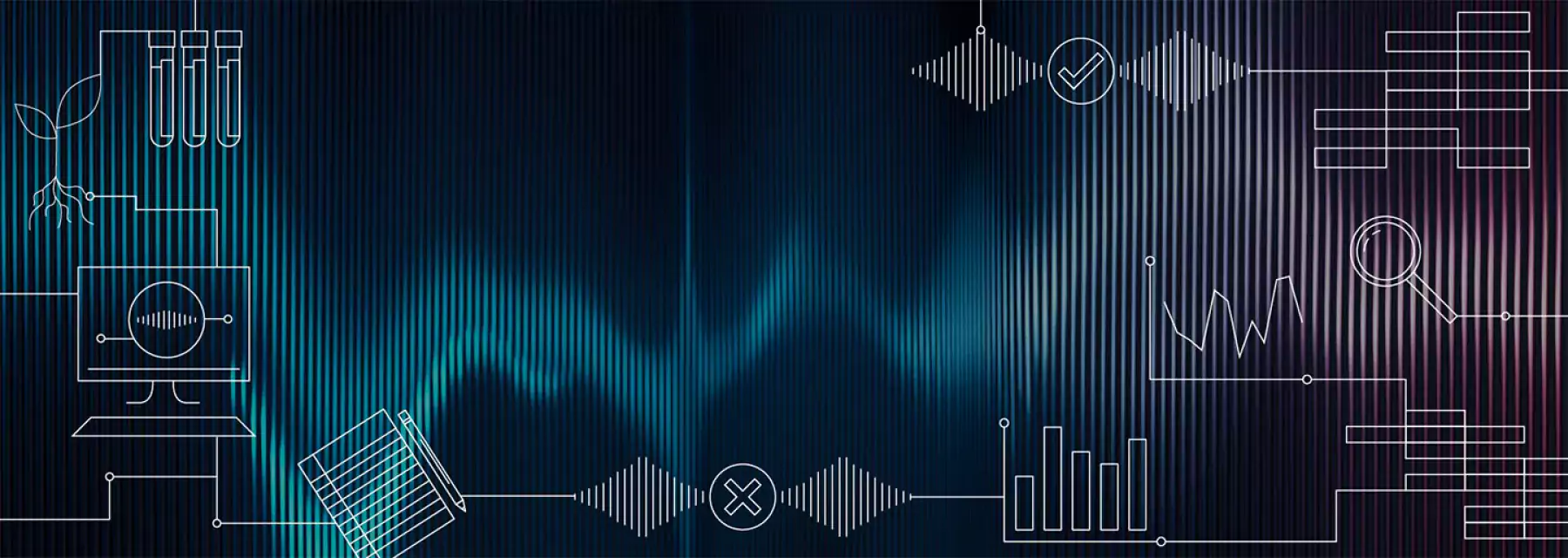
Anomaly Detection in Biosensor Waveforms
Student Capstone Project
MDS Okanagan students help Siemens Healthineers with new epoc device data pipelines
To help improve the identification of sensor failures within its epoc© Blood Analysis System, Siemens Healthineers, a leading medical technology company operating in over 70 countries with over 120 years of experience, and solutions that influence three-quarters of clinical decisions globally, enlisted the help of students from the UBC’s Master of Data Science Okanagan program to analyze biosensor timeseries waveform data.
Currently, specific time intervals are used to identify sensor failure throughout a sensor test. However, this method can be limited, particularly with sensors that have characteristically noisy waveforms. As a result, the detection of failure modes can contain a high false reject rate. Siemens Healthineers was therefore looking for help in developing an advanced system of failure identification that would offer improved classification of, and insight into, biosensor failures.
Enter the students from MDS Okanagan. Before applying any machine learning models, the students had to pre-process the signals by aligning them to a semantically consistent time point, making sure all the signals were of the same length, and performing noise reduction. The group then aimed to create an unsupervised machine learning pipeline that characterized unsuccessful readings into different groups so occurrences could be tracked over time. The tool would help identify possible root causes of failure which could then be addressed, improving the manufacturing process. To do so, the students tested and compared several methods for clustering on the unsuccessful readings with the aim of discovering hidden groupings in the types of sensor failures. In essence, they aimed to group the signals into similarly shaped readings.
Four MDS Okanagan students poured through tens of thousands of sensor waveforms. By first cleaning the data and then applying clustering algorithms, the students discovered several hidden clusters that characterized the sensor waveforms into different behaviours. In the end, the students delivered four promising pipelines that were successful to varying degrees, in clustering the sensor waveforms. The students also observed distinguishable shapes across the different clusters which could potentially be used in the future to identify and track root causes of sensor failure.
With the capstone project now complete, Siemens Healthineers now has four automated pipelines to aid in sensor waveform classification. In addition, the pipelines will serve as the starting point for classifying similarly behaving waveforms that can be investigated and potentially linked to common root causes of failure.
The project also provided the students with real-world experience in analyzing and communicating a client-focused problem.
Explore MDS Okanagan Explore Other Data in Action Stories