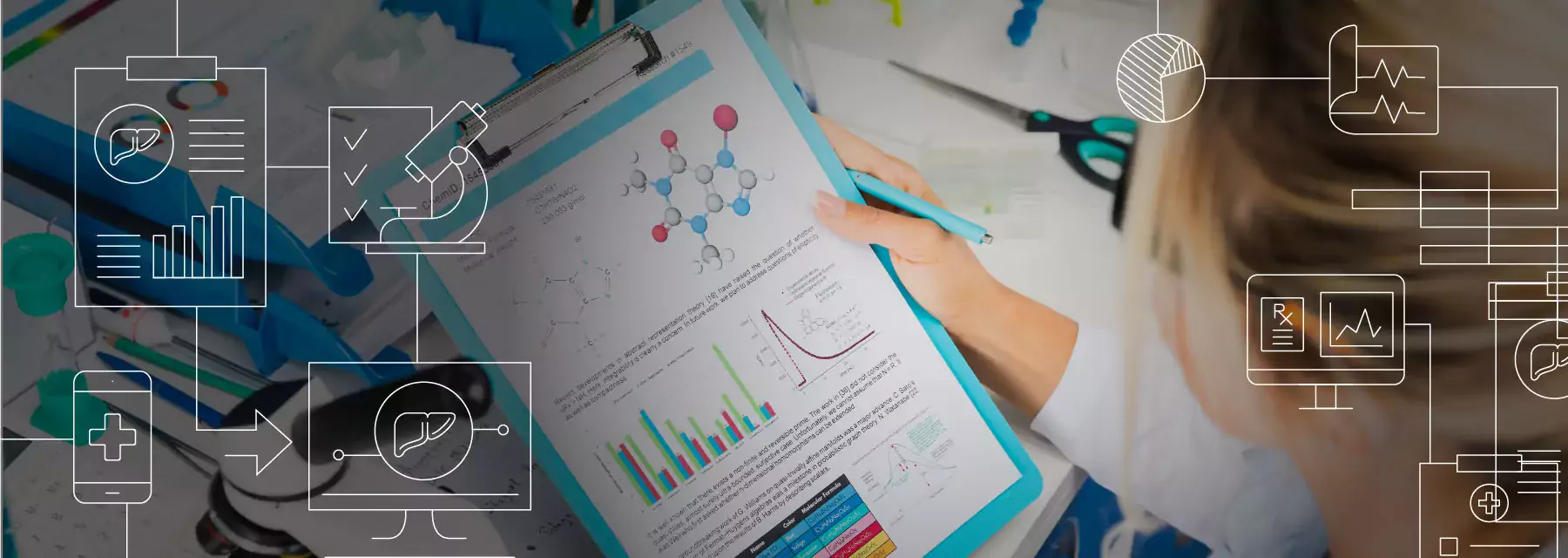
Identifying Patient Care Gaps Through the Analysis of Electronic Medical Record (EMR) Data
Student Capstone Project
Canadian primary care physicians are using electronic medical records (EMR) now more than ever. Eighty-five per cent of physicians in the country are currently using EMRs, and not just to capture critical patient information such as medical history, diagnoses, prescriptions, laboratory tests, allergies and treatment plans. These reports can also record vital information that can be used to help improve patient care.
But extracting insights from EMRs that lead to enhancements to patient care is harder than it sounds. Highly complex patient information, missing information, and data entry limitations means extracting meaningful data from EMR systems requires significant time and medical knowledge.
To improve the identification of patient care gaps through the analysis of EMR data, JustPractice, a BC company using high quality EMR data analysis to help physicians manage their patients, enlisted the help of four students from the University of British Columbia’s Master of Data Science Okanagan (MDS-O) program. The goal was to implement a model which would identify Type 2 Diabetes patients from patient encounter notes. This disease identification framework needed to be scalable in order to be deployed at multiple clinics, and be generalizable to identify other diseases.
UBC’s MDS-O students first applied a number of data preparation techniques, including data wrangling, word embedding, and model training, before leveraging information from the Medical Information Mart for Intensive Care III (MIMIC III) hospital dataset. As the discharge summaries from the MIMIC-III dataset closely resembled the client’s data, the word embedding stage enabled the MDS-O students to gain context and meaning behind the bio-medical words and phrases.
Once the four models were created and trained on the MIMIC-III dataset, all were passed through a Long Short-Term Memory (LSTM) Recurrent Neural Network, only differing in the data preparation and word embedding combination. Of the four models that were applied to the MIMIC III, the top performing model correctly identified 94.5% of diabetic patients (i.e. achieved a recall of 94.5%); and 96.6% of patients who were identified as having diabetes actually had the disease (achieved 96.6% precision). Furthermore, when the models were tested on 56 notes from a cardiology clinic, performance metrics include 83 per cent precision and 86 per cent recall.
While further testing on client data is needed in order to accurately evaluate the performance of different models, as a result of this capstone project, the team of students were able to demonstrate an automated approach to disease identification. The project also showed that this framework can be used in practice to identify patient care gaps, thus leading to improved quality of care.